Heart Damage Only in Vaxxed Kids? Unpacking Viral Claims
A Journal Club-Style Breakdown of a Recent COVID-19 Vaccine Study in Children
Earlier this week, pod co-host, Dr. Sarah Scheinman, wrote a great piece on how to read research studies. I (Jess) promised that I would do a follow-up from a data science perspective with a deep dive into research design red flags and ways to assess the validity of studies.
While working on it, I had at least a couple dozen people send me posts that have gone viral about a study that has, unfortunately, become fodder for anti-vaccine sentiment. I won’t reshare those posts here but the main refrain has been “MAJOR STUDY OF 1.7 MILLION CHILDREN: HEART DAMAGE ONLY FOUND IN COVID-VAXXED KIDS.” Scary stuff.
Note: We’ve done deep dives on myocarditis several times (in newsletters and many, many, many infographics)— but that isn’t the focus of this particular piece.
Instead, I thought this would be a great case study on how not to interpret research, one that might illustrate key study design and data concepts. Let's discuss.
The Study Deets
The study in question is a pre-print (not yet peer-reviewed) published in May of this year. It used data from the OpenSAFELY-TPP database in England to assess the effectiveness of two doses of Pfizer’s COVID-19 vaccine in kids. Specific adverse events (i.e., pericarditis and myocarditis) and overall safety (i.e., A&E attendance—aka ER visits—and unplanned hospitalization) were secondary outcomes.
Population: Children (5-11 years) and adolescents (12-15 years) in England
Sample Size: 1,643,258 total participants – 820,926 adolescents (410,463 vaccinated, 410,463 unvaccinated controls) and 566,284 children (283,142 vaccinated, 283,142 unvaccinated controls)
Vaccine: BNT162b2 (Pfizer-BioNTech)
Design: Observational study matching vaccinated individuals with unvaccinated controls
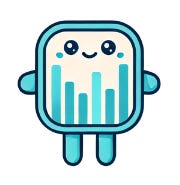
The study employed an observational design, specifically a matched cohort study, as opposed to an experimental design like a randomized controlled trial (RCT). In observational studies, researchers observe and analyze outcomes without controlling exposure (in this case, vaccination). By contrast, in experimental studies, such as RCTs, participants are randomly assigned to intervention groups.
Why is this distinction important? In observational studies, confounding factors—variables that influence both the likelihood of being vaccinated and health outcomes—can bias the results. For instance, individuals who are more likely to vaccinate may also have higher educational attainment, have higher socioeconomic status (SES), or engage in healthier lifestyle choices, all of which might be associated with better health outcomes. These factors could skew results in ways unrelated to the vaccine itself, thus clouding the relationship between vaccines and health outcomes which is what we are trying to isolate and measure.
In this study, researchers used matching (i.e., pairing vaccinated individuals with unvaccinated controls based on characteristics like age, sex, and region) to reduce some of these biases. However, residual confounding may still be present—factors like SES or specific health behaviors may still differ between the groups, leading to potential biases.
RCTs, on the other hand, reduce the impact of these confounding factors by randomly assigning exposures (e.g., who gets vaccinated and who doesn't). This process minimizes differences between groups from the start, making experimental studies better suited to support causal inferences. Observational studies, while valuable for real-world evidence and larger populations, are typically better for identifying associations rather than definitive cause-and-effect relationships.
RCTs can be more expensive and logistically challenging, so we often use observational studies as an alternative. Observational studies, especially those with large sample sizes that appropriately control for bias and confounding as much as possible, can still be quite powerful. They allow for large sample sizes and real-world data, they can assess rare outcomes that might be missed in smaller RCTs, and they can be useful when RCTs are impractical or unethical.
However, given their limitations, the results of observational studies should be considered alongside those from other study designs. Consistency across multiple observational studies can strengthen the evidence, but causal language should be avoided (remember, we are talking about associations). This design is common in vaccine safety studies, offering valuable insights while acknowledging inherent limitations in causal inference.
Okay, so what did the study find?
The study's findings can be categorized into three main areas: 1) vaccine effectiveness, 2) specific adverse events (myocarditis/pericarditis), and 3) overall safety.
The measure of effect calculated was the incidence rate ratio (IRR). The IRR is calculated by dividing the incidence rate in the exposed group by the incidence rate in the unexposed (or control) group.
Vaccine Effectiveness: The study found that vaccination was associated with a reduction in COVID-19-related healthcare utilization among adolescents:
COVID-19 A&E (emergency room) attendance was reduced by 40% in vaccinated individuals compared to unvaccinated (IRR 0.60, 95% CI 0.37-0.97).
COVID-19 hospitalization was reduced by 42% (IRR 0.58, 95% CI 0.38-0.89).
Formula: IRR = (Incidence Rate in Exposed [Vaccinated] Group) / (Incidence Rate in Unexposed [Unvaccinated] Group)
Interpretation:
An IRR of 1 indicates no difference in risk between the two groups.
An IRR greater than 1 suggests the event is more likely to occur in the exposed group.
An IRR less than 1 suggests the event is less likely to occur in the exposed group.
The study found that for COVID-19 A&E attendance, the IRR was 0.60 (95% CI 0.37-0.97).
This means vaccinated individuals (the exposed group) had 0.60 times the rate of COVID-19 A&E attendance compared to unvaccinated individuals (the unexposed group). In other words, vaccination was associated with a 40% reduction in the rate of COVID-19 A&E attendance (1 - 0.60 = 0.40 or 40%).
These results suggest that the vaccine was effective in preventing severe COVID-19 outcomes requiring medical attention. The confidence intervals (CI) indicate that we can be 95% confident that the true reduction in A&E visits lies between 3% and 63%, and for hospitalizations between 11% and 62%. This KEY TAKEAWAY— that the vaccines significantly reduced ER visits and hospitalizations— has conveniently been omitted from the posts that focus on the safety outcomes (more on those in just a sec).
First, I want to share a data science hot tip with you. When people hear the phrase “statistical significance,” the trusted “p-value” typically comes to mind.
As a refresher, a p-value represents the probability of obtaining results at least as extreme as those observed, assuming the null hypothesis is true. In other words, it's the likelihood of seeing the results we get (or more extreme ones) just by random chance if there were truly no effect. If the p-value is below a certain threshold (commonly 0.05), we consider the results statistically significant. This threshold corresponds to a 5% significance level, meaning we are accepting a 5% chance of mistakenly concluding that there's an effect when there isn't one (Type I error). Some fields, like economics, occasionally use a 90% significance level (or 0.10 p-value threshold), but 95% is the most common across disciplines.
But many people overlook confidence intervals (CIs)! A confidence interval gives us a range of values within which we believe the true effect lies. For example, a 95% confidence interval means that if we were to repeat the study multiple times, 95% of the time, the true effect would fall within that range.
Now, what we are looking for in a confidence interval is the null value—which represents no difference between the two groups being compared. The null value depends on the measure we're using. Often, in research, we set up ratios to compare groups. In this case, we're looking at IRRs (incidence rate ratios)—but we also often see odds ratios, risk ratios, and others.
So, what's the bottom line here? These ratios compare the outcome of interest in the experimental (or exposed) group versus the control group. If the two groups have the same outcome, our numerator (experimental group outcome) and denominator (control group outcome) would be equal. And what does any fraction equal when the numerator and denominator are equal? One. Therefore, the null value for any ratio is 1.
If a confidence interval for a ratio contains the null value of 1, the result is not statistically significant, because it suggests that the true effect could be that there's no difference between the two groups.
Let's look at the two confidence intervals from the study above. Neither contains the null value of 1, meaning both results are statistically significant. This is an important indicator in any research where we are measuring the relative effect between groups.
Lastly, it's worth noting that if we look at absolute measures of effect (like risk difference), the null value is zero. This is because we are looking at the difference between the outcomes of our experimental and control groups. And, as you know, any number minus itself equals zero, making that the null value in this case.
Okay, back to the study findings…
Specific Adverse Events (Myocarditis/Pericarditis): The study identified 12 cases of myocarditis or pericarditis out of over 400,000 vaccinated adolescents. This translates to rates of 27 cases per million after the first dose and 10 per million after the second dose. Notably, no cases were reported in the unvaccinated group.
Overall Safety:
There was no observed increase in overall A&E attendance or unplanned hospitalizations in vaccinated groups.
No COVID-19-related deaths occurred in any group, vaccinated or unvaccinated.
The heart of the controversy lies in the study's findings on myocarditis and pericarditis, so let's examine those results closely and put them into perspective.
The study identified 12 cases of myocarditis or pericarditis out of over 400,000 vaccinated adolescents. This translates to rates of 27 cases per million after the first dose and 10 per million after the second dose. Notably, no cases were reported in the unvaccinated group.
What’s getting picked up by folks trying to discredit the safety of vaccines is that this is a MAJOR SAFETY SIGNAL that indicates that the vaccines are causing cardiac issues in our kids. That’s quite a claim considering that the absolute risk is extremely low, affecting only about 0.003% of vaccinated individuals. To put this in perspective, you're about 333 times more likely to be injured in a car accident in any given year than to experience myocarditis after COVID-19 vaccination based on this study's findings. (Car accident injury risk: 1% annually or 10,000 per million according to the National Highway Traffic Safety Administration).
It's also important to compare these risks to those associated with COVID-19 infection itself. A study in JAMA Cardiology found the risk of myocarditis to be 0.146% following COVID-19 infection compared to 0.009% following vaccination. In other words, the risk of myocarditis from COVID-19 infection is about 16 times higher than from vaccination. (It's unclear how this study accounted for SARS-CoV-2 infections during the follow-up period, which is a big issue in my opinion.)
Moreover, when myocarditis does occur post-vaccination, it tends to be mild. A CDC study found that 95% of cases were mild, with a median hospitalization time of just 2 days. This aligns with the overall safety profile observed in our study, which found no increase in A&E attendance (ER visits) or unplanned hospitalizations in the vaccinated group.
Statistical v. Clinical Significance
When interpreting these findings, we must consider both statistical and clinical significance. Clinical significance refers to the practical importance of a research finding in real-world medical practice. It asks whether an observed effect is large enough to have meaningful implications for patient care or health outcomes, regardless of statistical significance.
While the difference in myocarditis rates between vaccinated and unvaccinated groups might be statistically significant (though the study doesn't provide confidence intervals or p-values for these specific outcomes), we must ask: Is this difference meaningful in real-world medical practice?
To answer this, we need to weigh the risks against the benefits. The study found significant reductions in COVID-19-related healthcare utilization among vaccinated adolescents:
A 40% reduction in COVID-19 ER visits (IRR 0.60, 95% CI 0.37-0.97)
A 42% reduction in COVID-19 hospitalization (IRR 0.58, 95% CI 0.38-0.89)
These are substantial benefits that directly impact public health and individual wellbeing. When considering these benefits alongside the very low risk of myocarditis or pericarditis, the balance appears to favor vaccination.
The Art of Responsible Research Interpretation
This case study illustrates a critical point in public health communication: the danger of sensationalized interpretations of complex research. The viral claim that "HEART DAMAGE ONLY FOUND IN COVID-VAXXED KIDS" is a gross misrepresentation that distorts and fails to contextualize the study's findings.
Key takeaways for interpreting research responsibly:
Understand study design: Recognize the strengths and limitations of observational studies versus RCTs. Well-designed observational studies can and do have a ton of value, but they can’t be used to make causal inferences in and of themselves.
Understand relative vs. absolute risk: While it might sound alarming that myocarditis was only found in vaccinated children, consider the absolute risk. The rate of 27 cases per million or 0.003% reveals how extremely rare this event is. Presenting only the relative risk (vaccinated vs. unvaccinated) without the context of absolute risk can be misleading.
Contextualize findings: Compare risks to everyday events and to risks from the disease itself. For example, the annual risk of being injured in a car accident (about 1% or 10,000 per million) is roughly 333 times higher than the observed risk of myocarditis after vaccination (0.003% or 30 per million). Also, consider that the risk of myocarditis from COVID-19 infection itself is significantly higher than from vaccination.
Look at the full picture: Don't cherry-pick data. This study showed significant reductions in COVID-19 hospitalizations among vaccinated youth. That ‘lil tidbit (which was the main takeaway from the study) has been omitted from every post I’ve seen shared on this study. If we're going to have an honest conversation about this topic, we have to look at and interpret the full body of evidence, not just those that align with our beliefs (aka confirmation bias).
No study is an island: This is a biggie. Public health decisions are based on comprehensive reviews of multiple studies, not single studies (especially those that have not yet gone through the rigorous peer review process). I’d be remiss if I failed to mention the many vaccine surveillance programs and systems in place that support the overall safety and effectiveness of these vaccines.
As consumers of information, we must approach research with critical thinking and context. This study, when properly interpreted, adds to the body of evidence supporting the safety and efficacy of COVID-19 vaccines in young people. However, it's just one piece of a larger puzzle.
In an era of rapid information spread, the ability to interpret research responsibly is not just an academic skill—it's a vital tool for public health. By understanding how to approach studies critically and in context, we can make more informed decisions about our health and contribute to more productive public health discussions.
Stay curious,
Unbiased Science